The Chinese Control Conference
Pre-conference Workshop 2
Learning control frontier
Speaker: Zhongsheng Hou, Qingdao University, China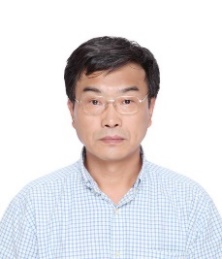
Title: How to design a control system with ability of utilizing data and knowledge?
Abstract: Professor R. E. Kalman was the founder and visionary leader of the field in modern control theory. His influence transcends well beyond system and control into diverse fields of engineering, mathematics, and others. However, there have been huge significant developments in science, engineering, technology, and society in the last few decades. It is clear that change will accelerate further in the coming decades. Thus, thinking about the relevance and framework of the control theory in post-Kalman under big data, IIoT or AI age, that might illuminate the path of the system and control research for the future.
This talk includes five parts. Background of big data/IIoT/AI; Kalman’s Paradigm and its Challenges; Model free adaptive control (MFAC) and its ability of utilizing data and knowledge; Relationships between MFAC with adaptive control and PID; and Conclusion.
Biography: Zhongsheng Hou (SM’13, F’20) received the B.S. and M.S. degrees from Jilin University of Technology, Jilin, China, in 1983 and 1988, respectively, and the Ph.D. degree from Northeastern University, Shenyang, China, in 1994. From 1997 to 2018, he was with Beijing Jiaotong University, Beijing, China, where he was a Distinguished Professor and the Founding Director of Advanced Control Systems Lab, and the Head of the Department of Automatic Control. He is currently a Chair Professor with Qingdao University, Qingdao, China.
His research interests are in the fields of data-driven control, model-free adaptive control, learning control, and intelligent transportation systems. He has authored two monographs, Nonparametric Model and its Adaptive Control Theory, Science Press (in Chinese), 1999, and Model Free Adaptive Control: Theory and Applications, CRC Press, 2013. His pioneering work on model-free adaptive control has been verified in more than 230 different field applications, laboratory equipment and simulations with practical background, including wide-area power systems, lateral control of autonomous vehicles, temperature control of silicon rod.
Prof. Hou is the Founding Director of the Technical Committee on Data Driven Control, Learning and Optimization (DDCLO), Chinese Association of Automation (CAA), and is a Fellow of CAA. Dr. Hou was the Guest Editor for two Special Sections on the topic of data-driven control of the IEEE Transactions on Neural Networks in 2011, and the IEEE Transactions on Industrial Electronics in 2017.
Speaker: Guangming Xie, Peking University, China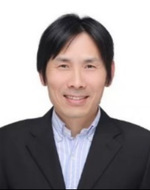
Title: From Simulation to Reality: A Learning Framework for Fish-like Robots to Perform Motion Control Tasks
Abstract: The fish-like robot has the advantage of high maneuverability with low noise due to its bio-inspired structure and biomimetic locomotion. However, it is challenging to efficiently design motion controllers for such robots to achieve satisfactory performance on specific control tasks in the real underwater environment, since the complex fluid-structure interaction exists during their swimming and exact dynamic models are absent. In this talk, we propose a learning framework, incorporating a simulation system and a training methodology, to autonomously and fast train in simulation to create control policies that are capable of directly applying to a type of physical fish-like robots to perform motion control tasks. First, we construct a simulation system combining a data-driven environment and a computational fluid dynamics (CFD)-based environment, thus well balancing the simulation accuracy and the calculation speed. Second, we design a training methodology to train deep reinforcement learning (DRL)-based policies for the robot in our constructed simulation system to perform a specific control task. Then, we use two typical motion control tasks to verify our proposed framework. One is the path-following control task which is a one-objective problem with dense rewards, while the other is the pose control task which is a two-objective problem with sparse rewards. For each task, the DRL-based control policy trained by our learning framework is directly deployed on the physical fish-like robot to perform the task in the real world. Experimental results show that the policies trained in simulation still work well in the real world, and perform even better in terms of control accuracy and stability compared with the traditional control methods, thus demonstrating the effectiveness of our learning framework.
Biography: Guangming Xie received his B.S. degrees in both Applied Mathematics and Electronic and Computer Technology, his M. E. degree in Control Theory and Control Engineering, and his Ph.D. degree in Control Theory and Control Engineering from Tsinghua University, China in 1996, 1998, and 2001, respectively. Then he worked as a postdoctoral research fellow in the Center for Systems and Control, Peking University, China from July 2001 to June 2003. Now he is a full Professor of Dynamics and Control in the College of Engineering, Peking University and the founder and director of the Intelligent Biomimetic Design Lab (IBDL), Peking University. He is the main editor of Mathematical Problems in Engineering and serves as associate editors of several international journals. His research interests include smart swarm theory, multi-agent systems, multi-robot cooperation, biomimetic robot, and networked control systems. He has published over 200 papers and three books. He is one of the Most Cited Chinese Researchers from Elsevier (Control and system engineering), from 2014 to 2021.
Speaker: Dongbin Zhao, Chinese Academy of Sciences, China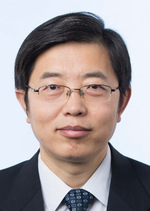
Title: Deep Reinforcement Learning based Game Decision Making
Abstract:Deep reinforcement learning (DRL) has laid several milestones in artificial intelligence (AI), as the DQN algorithm to conquer the Atari video games, AlphaGo for the most difficult board game Go, AlphaZero for more general board games, AlhpaStar for real-time strategy game Starcraft II, Muzero as a general game AI for both board games and video games, and Gato as a generalist agent for kinds of decision making problems. Recent years witnessed the rapid progress of DRL in different kinds of domains to become a very hot topic of research and applications. This talk will introduce the major achievements and recent progresses of DRL algorithms, and present some efforts on decision making for games and robotics from the speaker’s group, and pack up some possible future trends.
Biography:Dongbin Zhao is a professor with the Institute of Automation, Chinese Academy of Sciences, and the University of Chinese Academy of Sciences, China. Dr. Zhao serves as the Associate Editor of IEEE Computational Intelligence Magazine, IEEE Transactions on Cybernetics, IEEE Transactions on Artificial Intelligence, IEEE Transactions on Cognitive and Developmental Systems (TCDS), etc. He is the Chair of Strategy Planning Committee of IEEE Computational Intelligence Society. He is involved in organizing many international conferences, including General Chair of IEEE Conference on Games 2022. He has published 6 books, and over 300 international journal and conference papers, including the 2022 Best Paper Award of IEEE TASE, 2022 Outstanding Paper Award of IEEE TETCI, and 2020 Outstanding Paper Reward of IEEE TCDS. His group won over 10 first prizes in AI competitions, including 3 championships of 2020 RoboMaster AI Challenge, and the Championship for Fighting AI Competition of 2020 IEEE Conference on Games. His current research interests lie in deep reinforcement learning, computational intelligence, smart driving, game artificial intelligence, robotics, etc. He is an IEEE/CAA/AAIA Fellow.
Speaker: Jing Na, Kunming University of Science & Technology, China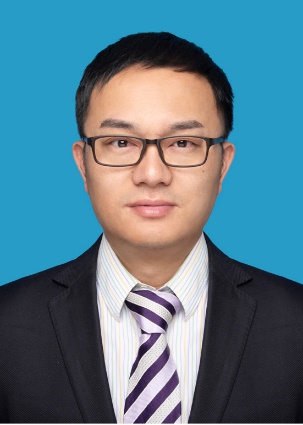
Title: Adaptive Parameter Estimation and Control via Estimation Error: Theory and Application
Abstract: Adaptive parameter estimation and adaptive control have been well developed to achieve modeling and control for uncertain systems. However, the well-known parameter estimation and adaptive control methods have been mainly designed based on the gradient algorithms (with appropriate modifications) by using the prediction error or control error. Hence, the parameter estimation convergence and the online verification of the required persistent excitation (PE) condition are generally difficult with this framework. In this talk, we will introduce a novel robust, fast adaptive parameter estimation framework, where the estimation error between the unknown parameters and their estimates are explicitly obtained and then use to drive online adaptation algorithms. This new adaptation even allows to achieve finite-time parameter estimation, and can be easily incorporated into adaptive control designs to achieve tracking control and parameter estimation simultaneously. We will also introduce an intuitive and numerically feasible approach to online verify the PE condition. Finally, several practical application of this new adaptation to in-car parameters estimation, ITER cryogenics system identification, and adaptive control for robotics, wave energy converters (WECs) and other realistic systems will be presented.
Biography: Jing Na is currently a Professor with the Faculty of Electrical & Mechanical Engineering at Kunming University of Science & Technology. He received the B.S. and Ph.D. degrees from the School of Automation, Beijing Institute of Technology, China, in 2004 and 2010, respectively. From 2011 to 2012, he was a Monaco/ITER Postdoctoral Fellow with the ITER Organization, France. From 2011 to 2012, he was a Marie Curie Fellow with the University of Bristol, UK. He has hold also visiting positions with the Universitat Politecnica de Catalunya, Spain, and with the University of Bristol, UK.
His current research interests include parameter estimation, adaptive control, and nonlinear control with application to vehicle systems, servo mechanisms and energy conversion plants (e.g., engine, wave energy convertors, etc.). He has authored/co-authored more than 100 peer reviewed journal and conference papers. Dr Na has been awarded the Best Application Paper Award of the 3rd IFAC International Conference on Intelligent Control and Automation Science (IFAC ICONS 2013), and the 2017 Hsue-shen Tsien Paper Award.
He is currently an Associate Editor of the IEEE Transactions on Industrial Engineering, and Neurocomputing. He has also served as an international program committee Chair of ICMIC 2017 and DDCLS 2019 and IPC member of many international conferences. He has organized several special issues in international journals, and invited session in several prestigious conferences.